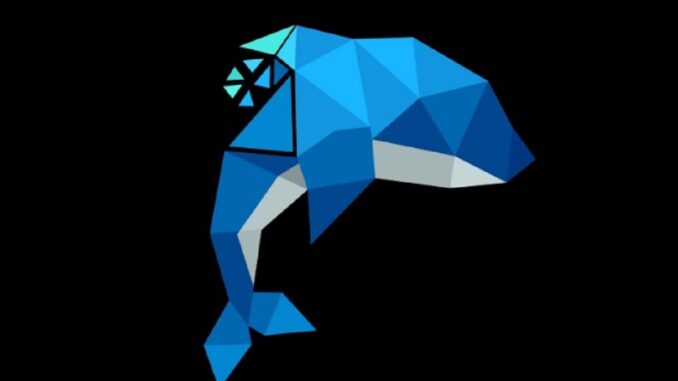
Microsoft AI has recently announced a new model called ORCA (Optimizing Reasoning with Common Sense and Attention), which aims to improve the reasoning abilities of large foundation models (LFMs) such as GPT-3 and T5.
ORCA is a 13-billion parameter model that leverages a large-scale common sense knowledge base and an attention mechanism to generate natural language explanations for various reasoning tasks.
What are LFMs and why do they need reasoning?
LFMs are pre-trained language models that can perform a wide range of natural languages understanding and generation tasks, such as answering questions, summarizing texts, writing essays, and more.
LFMs are trained on massive amounts of text data from the web and learn to capture general linguistic patterns and knowledge. However, LFMs often struggle with tasks that require logical reasoning, common sense, or domain-specific knowledge. For example, LFMs may fail to answer questions that involve multiple steps of inference or generate texts that are inconsistent or implausible.
How does ORCA work?
ORCA is designed to enhance the reasoning capabilities of LFMs by learning to imitate their reasoning process and generate natural language explanations for their outputs. ORCA consists of two main components: a knowledge retriever and a knowledge explainer.
The knowledge retriever uses a transformer-based encoder to query a large-scale common sense knowledge base called ConceptNet, and retrieve relevant facts that can support the reasoning task. The knowledge explainer uses another transformer-based decoder to generate natural language explanations based on the retrieved facts and the input query. ORCA can be applied to any LFM as a plug-and-play module, without modifying the original LFM.
What are the benefits of ORCA?
ORCA has several advantages over existing methods for improving the reasoning abilities of LFMs. First, ORCA can generate natural language explanations that are not only informative but also interpretable, which can help users understand how the LFM arrived at its output and whether it is trustworthy.
Second, ORCA can leverage external knowledge sources such as ConceptNet, which can provide rich and diverse common sense facts that are often missing or implicit in the text data used to train LFMs. Third, ORCA can adapt to different reasoning tasks and domains by changing the query format and the knowledge base, without requiring additional training or fine-tuning.
What are some applications of ORCA?
ORCA can be used for various natural language understanding and generation tasks that require reasoning, such as question answering, text summarization, text generation, and more.
For example, ORCA can help users find answers to complex questions that involve multiple steps of inference or common sense knowledge, such as “Why do people wear sunglasses?” or “How can you prevent a fire?”. ORCA can also help users evaluate the quality and credibility of the texts generated by LFMs, such as summaries, essays, or stories, by providing natural language explanations that highlight the relevant facts and logic behind them.
Leave a Reply